A vibrant menagerie of email classifiers
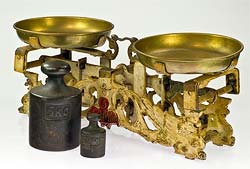
If you look through my Definitive Guide to Information Overload Solutions you will see an entire chapter dedicated to automation of incoming email classification – that is, software solutions that classify incoming messages by a variety of attributes to achieve two main goals: prioritize messages that are important to the recipient over those who are not, and aggregate messages into groups of a common nature.
These solutions form a collection which is a wonder to behold. They define the outcome of message classification from a great many angles, including:
- Prioritizing messages by the assessed urgency of the required processing (the concept used in Knowmail).
- Clustering messages by deduced importance (done in KeyMails, ClearContext and SaneBox).
- Clustering by content category (e.g. Advertising, Travel, Social, Finance) and presenting them in separate “stacks”: (like AOL Alto and Inky).
- Clustering by user-selected parameters like subject, size, sender, age, or source type (Mailstrom).
The main challenge for all these tools is to read the mail and understand what it says. Thirty years ago you’d need a human assistant to do that – these days we have computers. Different solutions do it in different ways, varying from quite simple rules to the bleeding edge of semantic analysis (the term AI is out of favor, but that’s exactly what’s involved). For example, we see methods that:
- Focus on messages from contacts you’ve interacted with in the past via email (Swingmail) or social media (Cloze).
- Analyze your past actions, as well as interconnections between email senders, to rank incoming emails by importance (EmailTray).
- Rely on indications of important or actionable messages provided by the sender (Hiri and Inbox Pro).
- Allow users to explicitly or implicitly indicate importance by example, thereby training the tool over time (Gmail’s Priority Inbox and others).
- Use a combination of automated software and remote human assistants (ZeroMail).
This list captures just part of the diversity in classification concepts we see – it’s truly a fascinating menagerie of ingenious applications!
How do we assess these solutions?
Of course, the immediate question becomes:
How well do these tools do their thing? Do they really discern the importance (or class) of each message?
Actually, as with any classifier, there are two issues here: the frequency of False Positives – when the tool says something is important, and it isn’t – and of False Negatives – when it misses something important.
These are important parameters, because these tools ask us to entrust to them our main method of communications – and our allocation of our time to handle our interactions with people. This can be a big deal, and it’s easy to imagine horror stories when people lose their jobs, or a lottery win, because of a False Negative (though in all fairness, with hundreds of incoming mails a day, missing important messages can and does happen even without any classifier).
So – we need an objective measure, a benchmark, of classifier performance, not only to help us choose which classifier is best, but also to decide which one is best for each of us – there may well be tradeoffs that vary from user to user.
And there is no such objective measure available at this time.
A call to action
I propose that we need a standard industry benchmark of the accuracy and effectiveness of email classifier tools. This benchmark should specify how to measure tool performance, and should provide a standard set of messages to be used in this assessment. Actually the set of messages is easy – we have the creepy Enron Database available to us. What we need is a procedure and set of measures, and of course we need agreement by many vendors and researchers that this is the standard we believe in.
So – a challenge to you all: anyone willing to initiate the definition of such a benchmark?
Image courtesy Nikodem Nijaki, via Wikimedia Commons.